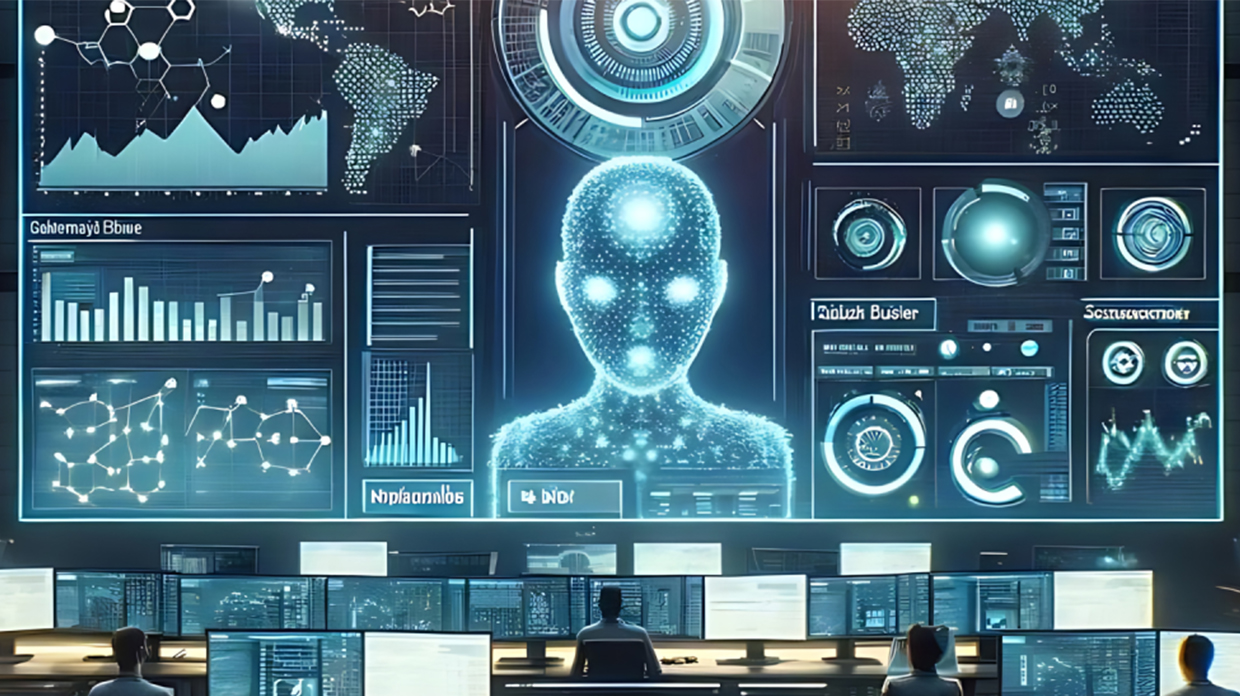
How AI Works in Banking Fraud Detection: A Complete Guide
Artificial Intelligence (AI) is revolutionizing banking by providing robust tools for fraud detection. Financial institutions are using AI algorithms to analyze massive amounts of transactional data in real time, identify anomalies, and predict fraudulent behavior. This guide will explore how AI works in banking fraud detection and its transformative impact on financial security.
What is Banking Fraud Detection?
Banking fraud detection involves identifying and preventing unauthorized financial transactions that can harm customers and institutions. Common types of banking fraud include:
-
Credit Card Fraud: Unauthorized card transactions
-
Identity Theft: Using stolen identities to access accounts
-
Account Takeover: Gaining control of customer accounts
-
Money Laundering: Illicitly concealing money origins
-
Phishing Attacks: Deceptive methods to steal sensitive information
How AI Detects Fraud in Banking
-
Behavioral Analysis and Pattern Recognition
AI algorithms analyze historical customer data to create unique behavioral profiles. This includes transaction amounts, frequency, locations, and device information. When a transaction deviates from normal behavior, it triggers alerts.
-
Example: A customer typically spends $100 daily but suddenly spends $10,000. AI flags this anomaly.
-
Real-Time Transaction Monitoring
AI can monitor millions of transactions in real time, identifying suspicious patterns instantly. It filters false positives and raises alerts for genuine threats.
-
Example: Detecting multiple failed login attempts from different locations in quick succession.
-
Machine Learning and Predictive Analytics
AI models learn from past fraudulent activities to enhance prediction accuracy. They adapt and evolve over time, making them resilient to new fraud techniques.
-
Example: AI predicts fraud based on past behaviors, reducing false positives.
-
Natural Language Processing (NLP)
NLP helps AI understand text-based fraud attempts, such as phishing emails and scam messages. It analyzes email content, keywords, and sender authenticity.
-
Example: Detecting suspicious phrases in phishing emails.
-
Identity Verification Using Biometrics
AI-driven biometric tools verify identities through facial recognition, fingerprints, and voice analysis, preventing unauthorized access.
-
Example: Mobile banking apps using face recognition to verify users.
Advantages of AI in Banking Fraud Detection
-
Speed and Efficiency: Real-time analysis reduces response time.
-
Accuracy and Precision: Reduces false positives and accurately detects fraud.
-
Scalability: Manages large volumes of data seamlessly.
-
Adaptability: Learns from new fraud patterns.
-
Cost-Effective: Reduces manual intervention and operational costs.
Challenges of AI in Banking Fraud Detection
-
Data Privacy Concerns: Handling sensitive customer data requires strict compliance.
-
Evolving Fraud Techniques: AI must adapt to emerging fraud methods.
-
High Implementation Costs: Initial setup can be costly.
-
False Positives: Incorrectly flagging legitimate transactions can disrupt customers.
Future of AI in Banking Fraud Detection
AI will continue evolving with advancements in deep learning, predictive analytics, and quantum computing. The future will bring even smarter fraud detection tools, integrating AI seamlessly into financial services.
Conclusion
AI is revolutionizing fraud detection in banking by providing real-time, accurate, and scalable solutions. Its ability to adapt and learn from evolving threats ensures financial institutions stay one step ahead of fraudsters. With continuous advancements, AI will remain a cornerstone of banking security.